"Our AI model identifies almost uncorrelated alpha sources"
Selected systematic and fundamental equity strategies in Zürcher Kantonalbank's Asset Management division have recently been supported by an AI model. Stefan Fröhlich and Fabian Ackermann explain what artificial intelligence can achieve.
Interview with: Stefan Fröhlich, Senior Portfolio Manager and Fabian Ackermann, Head of Systematic Strategies
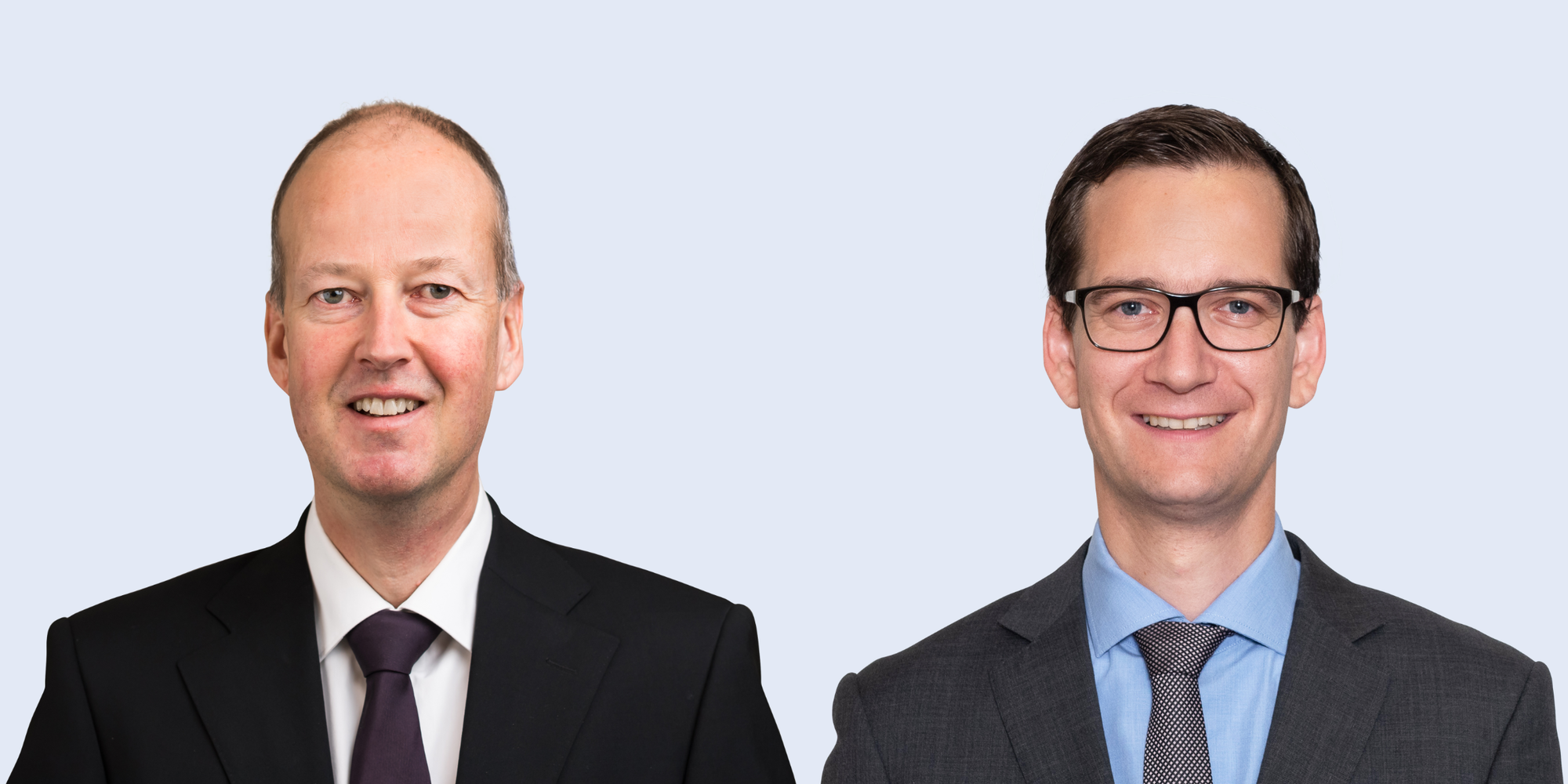
Stefan, why do we need another approach with artificial intelligence in addition to the classic fundamental and systematic investment approaches?
Equity markets are complex and are influenced by a variety of factors such as corporate earnings, macroeconomic data, geopolitical events and technological developments. There is no one right strategy for equity strategies, but rather many promising approaches, each of which utilises different alpha sources and all of which have their justification. Our AI model identifies almost uncorrelated alpha sources. The key is to find the various alpha sources, know their risk profile and combine them skilfully.
What added value does the additional integration of your AI model bring?
AI is free of prejudices and emotions such as greed, fear, panic or herd instinct. Our model also has a perfect memory for the respective training period. We humans, on the other hand, have a selective memory and can only vaguely remember events that happened far in the past. In addition, young fund managers do not yet have many years of experience with various economic cycles, stock market crashes and recessions. The fact that the AI model adapts dynamically to changing market conditions means that trends and opportunities are also recognised quickly. The model can also analyse large volumes of data within a very short space of time, which would be almost impossible for humans to handle.
What does that mean in concrete terms?
Here is an example: the MSCI World Small Cap Index is broadly diversified and contains over 4,000 stocks. With a fundamental investment approach, it would take an estimated dozen analysts and fund managers to cover this universe. Our AI model and our machine learning algorithm can efficiently and comprehensively cover all equities worldwide.
AI characteristics at a glance
AI characteristics at a glance
Are you now delegating everything to the machine learning model?
Absolutely not. It goes without saying that we remain true to our investment philosophy, which we have lived by for over 20 years. Our decisions must always be comprehensible as well as economically and empirically sound. As a first step, we are integrating the machine learning model into selected strategies and will evaluate the development on an ongoing basis. We will continue to pursue our consistent and multifaceted risk management across all strategies. The majority of strategies remain both country- and sector-neutral and are managed against the respective benchmark with a target tracking error, which ultimately limits stock bets. The AI model provides additional alpha scores. Asset management is carried out as usual by portfolio managers. Sustainability criteria will also continue to be taken into account in all strategies.
Fabian, can you briefly describe what the investment process with artificial intelligence looks like in Asset Management at Zürcher Kantonalbank?
Our investment process primarily follows three steps. It starts with our stock database, which currently contains 7,000 stocks with data that has been updated daily for 25 years. We have collected over 500 company key figures per share, which can have an influence on future returns (alpha sources). This raw data is a kind of training ground for our machine learning algorithm. The algorithm learns independently from the processed data, recognises patterns and uncovers hidden correlations between company key figures and future returns. We then aggregate the predictions of several algorithms to obtain daily return forecasts for all shares worldwide.
The third step is to construct the portfolios?
Correct. We use the risk model integrated into our portfolio management system for this purpose. It helps us to maximise future returns and calculate the optimal portfolio. We also control risk, for example by minimising sector and country bets and not making large bets on individual securities.
What can we imagine by "hidden connections"?
It is not trivial to understand why our machine learning model favours a stock or not. However, there are techniques that can be used to take a look inside our complex model. This is where the so-called SHAP values (SHapley Additive ExPlanations) come into play. They measure the contribution of each element of a return forecast, i.e. the alpha score. Thanks to the integration of SHAP values into our analysis process, we gain a deeper understanding of which factors influence the return forecast and to what extent. It is important to bear this in mind: If the model was trained on distorted or erroneous data, the SHAP values also reflect these distortions.
What else can the model do?
Our machine learning model can also recognise non-linear effects. This can mean, for example, that our AI model assesses shares in the eighth or ninth decile of shareholder yield as attractive. However, it does not favour shares in the tenth decile, i.e. those with the highest shareholder yield. This is contrary to the value factor, which assumes that attractiveness increases constantly, i.e. linearly, with the level of shareholder yield.
Stefan, what do the previous backtests with the additional integration of AI in equity strategies show?
The machine learning model delivered added value in different economic phases. It has been shown that the alphas achieved show a low correlation with those of the existing main factors of value, quality and momentum. This was an important point that prompted us to integrate the machine learning model into certain strategies.
Be honest: baking tests are often better than reality.
Backtesting financial data is a science in itself and requires a great deal of expertise and experience. After a "live track record" of 20 years in selected strategies, we can confirm that the returns in reality are actually often somewhat lower than in backtesting. This can have various causes. For example, there may be a selection bias. We are reassured by the fact that the machine learning algorithm only ever had access to the data that was available at the time during backtesting. This is only possible to a limited extent with classic backtesting.